How machine learning can support fraud detection in online payments
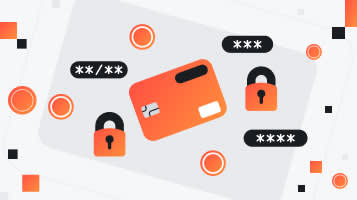
- •The scale of online payment fraud
- •Online payment fraud detection methods
- •How machine learning works in online payment fraud detection
- •Benefits of using machine learning in payment fraud detection
- •Considerations for machine learning to improve payment fraud detection
- •How Airwallex can help with payment fraud detection
The pandemic catalyzed the digitization of many businesses and changes in customer shopping behavior. Through this, digital payment transaction volume grew by 25 percent globally between 2019 and 2020.¹ While the growth in online transactions has had many benefits for businesses, it has also raised a new challenge: increased online fraud.
The ripple effect of a single fraudulent incident can be far-reaching. It affects both customers and your business, which is why fraud detection is important for proactive payment security. Artificial intelligence (AI) technology, like machine learning (ML), can identify and prevent real-time fraud attempts, helping safeguard global transactions, protect customers from payment fraud, and ensure long-term business success.
The scale of online payment fraud
As more businesses accept digital payments, online payment fraud is growing. Losses are projected to exceed $343 billion by 2027² due to activities like credit card fraud, identity theft, malware, and phishing.
The impact of online fraud on your business can be devastating, not only in terms of financial losses but also for your brand reputation. It can directly impact your bottom line through lost revenue from fraudulent transactions, chargebacks, and legal fees.
For customers, the impact is just as severe. They can lose money, have their personal information stolen, and lose trust in your brand. When customers fall victim to fraud, they may lose confidence in transacting with your business in the future and be wary of making online payments in general.
Using strong payment security measures and advanced fraud detection methods is important to protect your customers' financial information and avoid payment fraud.
Stop fraud before it occurs. Integrated payment fraud detection.
Online payment fraud detection methods
Traditional online fraud detection methods rely on rule-based systems, manual reviews, and behavioral analytics to find fraudulent activity.
The rule-based system involves setting specific rules to flag suspicious transactions within the payment processing system. Analysts manually review the transaction to determine if fraud is present. Behavioral analytics, which tracks patterns in user behavior, also detects unusual activity that may indicate fraud.
While traditional fraud detection methods offer a layer of protection, they can lead to false positives – errors that indicate fraud when it’s not present. This can cause legitimate transactions by your customers to be flagged for review before they can be completed. False positives require additional manual reviews by analysts, which can take some time. This leads to delays in processing real payments, causing frustration for your customers and potentially resulting in lost revenue.
More recently, machine learning has risen as an advanced method of fraud detection. ML can analyze huge volumes of transaction data in real time without an analyst, providing scalable fraud detection capabilities. It can quickly identify anomalies that traditional rule-based systems may miss. No matter how many transactions your business handles, machine learning can adapt to manage the growing volume efficiently.
How machine learning works in online payment fraud detection
Each time an online transaction is attempted, fraud detection systems work behind the scenes.
Machine learning quickly analyzes the historical transaction data of the card and compares it to the current transaction, looking at factors like the amount (is this transaction higher than normal?), the location (is the purchase being attempted from an unusual location?), the device and IP address (are these consistent?), and the purchase frequency (is the card being used to make multiple purchases in a short amount of time?), as well as many other factors. The model flags the transaction as suspicious in real time if any unusual patterns or behaviors are discovered. The transaction may fail immediately depending on the system or direct the cardholder to complete further verification checks.
Machine learning models are continuously learning and adapting, improving their ability to recognize and flag new fraud tactics as they appear. By analyzing feedback from incoming fraud reports and incorporating this knowledge into their algorithms, ML models become increasingly sophisticated in identifying and preventing fraudulent activity, helping ensure the online shopping experience remains safe and secure for your customers.
Benefits of using machine learning in payment fraud detection
Machine learning supports payment fraud detection by identifying patterns and uncovering unusual behavior as it takes place. Over time, these models improve their accuracy and effectiveness by continuously learning and adjusting to new patterns and scenarios, refining their understanding of what’s considered normal behavior versus what’s considered suspicious.
No matter the volume of transactions your business processes, ML systems can handle the load without compromising performance or security. They can scale up or down as needed, ensuring your fraud detection is still effective.
Machine learning also reduces the number of false positives over time, meaning fewer legitimate transactions are incorrectly flagged, improving the customer experience and decreasing the time analysts spend in review.
Considerations for machine learning to improve payment fraud detection
Prioritizing a strong payment fraud detection strategy is essential to safeguarding your business and protecting against fraud. Integrating rule-based fraud detection systems with machine learning models can help identify a broader range of fraudulent activities.
Since models learn from the data they’re given, unintended biases can sometimes form. For example, if the ML model is trained with only historical data from urban areas with high transaction volumes, the system might consider any transaction originating from a rural area as fraudulent, leading to unnecessary declines. To minimize unintended biases and promote fair fraud detection, it’s important to use diverse training data that represents a wide range of transaction scenarios and demographics.
User experience and privacy must also be balanced with fraud detection measures. While strict fraud detection measures are necessary, they shouldn't inconvenience real customers or compromise their privacy. Implementing frictionless authentication methods like facial recognition or a fingerprint scan can enhance security without significantly impacting the customer’s experience as their identity is verified.
Payment processors that use ML for advanced fraud detection are aware of these considerations and proactively work to resolve them. For example, Airwallex runs regular quality checks to ensure accuracy and uses seamless authentication methods to help protect customer privacy. Customers benefit from advanced fraud protection without having to manage these challenges themselves.
How Airwallex can help with payment fraud detection
Airwallex’s global Payments solutions use machine learning-powered fraud detection systems to improve the security of your online payments. Our fraud prevention engine efficiently identifies and blocks suspicious activity by analyzing transaction patterns in real time. This proactive approach not only helps safeguard your business from fraud but creates a more reliable payment experience for your customers, driving loyalty and online sales.
Grow and protect your global revenue.
Sources:
¹https://www.mckinsey.com/industries/financial-services/our-insights/sustaining-digital-payments-growth-winning-models-in-emerging-markets
²https://www.juniperresearch.com/press/online-payment-fraud-losses-to-exceed-343bn/
Share

Erin is a business finance writer at Airwallex, where she creates content that helps businesses across the Americas navigate the complexities of finance and payments. With nearly a decade of experience in corporate communications and content strategy for B2B enterprises and developer-focused startups, Erin brings a deep understanding of the SaaS landscape. Through her focus on thought leadership and storytelling, she helps businesses address their financial challenges with clear and impactful content.
View this article in another region:AustraliaNew ZealandGlobal
Related Posts
Is Airwallex safe?
•10 minutes

Payment methods guide: Navigating options for US businesses
•10 mins